Estudio: Consumo Energético ChatGPT
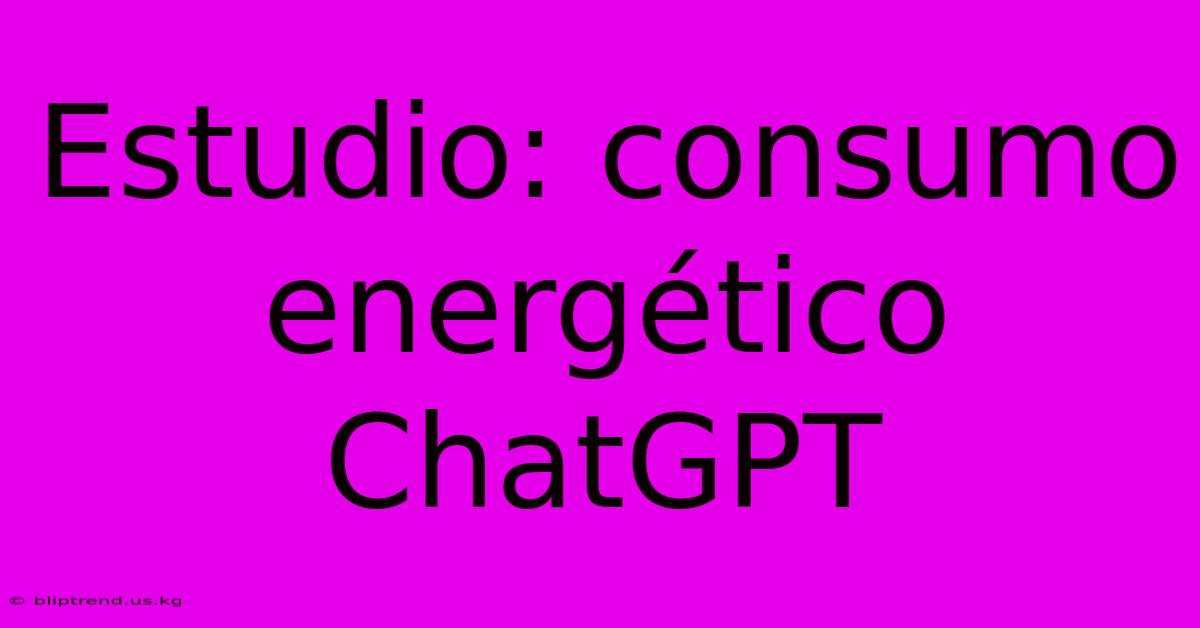
Discover more in-depth information on our site. Click the link below to dive deeper: Visit the Best Website meltwatermedia.ca. Make sure you don’t miss it!
Table of Contents
Decoding ChatGPT's Energy Consumption: A Deep Dive into the Environmental Impact of Large Language Models
Introduction: Dive into the surprisingly complex world of ChatGPT's energy consumption. This detailed exploration offers expert insights and a fresh perspective on the environmental footprint of large language models (LLMs), a topic of growing concern for both tech enthusiasts and environmental advocates. We'll unpack the multifaceted nature of this energy demand, exploring the factors driving it, the ongoing efforts to reduce it, and the broader implications for the future of AI.
Hook: Imagine the sheer computational power required to process billions of words, understand nuanced queries, and generate human-quality text—that's the reality powering ChatGPT. This seemingly effortless interaction hides a significant energy consumption, raising crucial questions about sustainability and the environmental impact of this rapidly advancing technology.
Why It Matters: The energy consumed by ChatGPT and similar LLMs is not just a technical detail; it's a critical factor influencing the broader adoption and responsible development of AI. Understanding this energy footprint is essential for mitigating its environmental impact and ensuring the sustainable growth of the AI industry. High energy consumption contributes to greenhouse gas emissions, exacerbating climate change. Furthermore, the cost of this energy impacts the accessibility and affordability of these powerful tools.
In-Depth Analysis: The energy consumption of ChatGPT is a complex issue, influenced by a multitude of intertwined factors. Let's break down the key contributors:
-
Model Size and Complexity: The sheer size of the LLM directly impacts energy consumption. ChatGPT, being a large language model, requires vast amounts of computational resources for training and operation. Larger models, with more parameters, demand significantly more energy. This is because more parameters mean more calculations are needed for every interaction.
-
Training Process: The training phase is particularly energy-intensive. This involves feeding the model massive datasets, requiring immense computational power across numerous powerful GPUs (Graphics Processing Units) running for extended periods. The energy used during training can dwarf the energy used for subsequent inference (generating text in response to prompts).
-
Inference (Generating Responses): While less energy-intensive than training, the inference process still consumes considerable energy. Each user query triggers a series of complex calculations within the model, demanding resources from powerful servers. The number of concurrent users directly influences the overall energy consumption.
-
Data Center Infrastructure: The infrastructure supporting ChatGPT's operation, including data centers, plays a crucial role in its energy consumption. Data centers require significant energy for cooling, power distribution, and maintaining optimal operating conditions. The efficiency of these data centers significantly influences the overall environmental impact.
-
Hardware Efficiency: The efficiency of the hardware used (GPUs, CPUs, and networking equipment) directly impacts energy consumption. Advances in hardware technology are constantly improving efficiency, but the scale of LLMs often pushes the boundaries of current capabilities.
Breaking Down the Essence of ChatGPT's Energy Consumption
Key Aspects to Explore:
-
Purpose and Core Functionality: Understanding the fundamental processes behind ChatGPT's operation—from tokenization to attention mechanisms—provides a foundation for comprehending its energy demands. Each step requires computational resources.
-
Role in Data Processing: The volume and complexity of data processed by ChatGPT significantly impact energy use. Processing large datasets necessitates substantial computational power, contributing to the overall energy footprint.
-
Influence on Server Load: The number of simultaneous users directly correlates with server load and, consequently, energy consumption. Peak usage periods will naturally lead to higher energy demand.
-
Algorithmic Optimization: The algorithms used to train and operate ChatGPT play a vital role. More efficient algorithms can significantly reduce energy consumption without sacrificing performance.
Exploring the Depth of ChatGPT's Energy Impact
Opening Statement: The environmental impact of ChatGPT is a complex issue, extending beyond simple energy consumption. It encompasses the entire lifecycle of the technology, from manufacturing hardware to the eventual disposal of equipment.
Core Components: We need to consider the entire life cycle assessment (LCA) of ChatGPT's infrastructure. This includes:
-
Hardware Manufacturing: The production of GPUs and other hardware components requires energy and resources, generating significant emissions.
-
Data Center Operations: Data centers' energy consumption includes not only electricity but also the water used for cooling, and the potential environmental impact of waste heat.
-
Transportation and Logistics: The transportation of hardware and components adds to the overall carbon footprint.
-
End-of-Life Management: The disposal of obsolete hardware raises concerns about e-waste and its environmental consequences.
Relation Exploration: Let's examine the relationship between specific factors and ChatGPT's energy consumption. For example:
Subheading: Enhancing Algorithmic Efficiency Within the Framework of ChatGPT's Energy Consumption
Overview: Optimizing algorithms is crucial for reducing the energy demands of LLMs. This involves developing more efficient training methods and inference processes.
Key Details: Research into techniques like model quantization (reducing the precision of calculations), pruning (removing less important connections), and knowledge distillation (training smaller models to mimic larger ones) offers promising paths toward energy efficiency.
Integration: These algorithmic improvements can be integrated seamlessly into the existing ChatGPT architecture, leading to considerable reductions in energy consumption without significant performance degradation.
Insight: By focusing on algorithmic efficiency, we can significantly reduce the environmental impact of ChatGPT and similar LLMs without compromising their capabilities.
FAQs for ChatGPT's Energy Consumption:
-
What is the total energy consumption of ChatGPT? Precise figures are difficult to obtain due to the proprietary nature of the technology and the distributed nature of its infrastructure. However, research suggests it's substantial.
-
How can I reduce my contribution to ChatGPT's energy consumption? Using ChatGPT more efficiently (avoiding unnecessary requests, using concise prompts) can indirectly contribute to lower overall energy demand.
-
What is being done to reduce the energy consumption of LLMs? Researchers are actively exploring various techniques, including those mentioned above, aiming to create more energy-efficient LLMs.
-
Will future LLMs be more energy-efficient? Continuous advancements in hardware and algorithmic efficiency strongly suggest future LLMs will have a significantly lower energy footprint.
Tips for Minimizing the Environmental Impact of Using ChatGPT:
-
Master the Basics: Use clear and concise prompts to minimize the computational effort required to generate responses.
-
Step-by-Step Guide: Break down complex requests into smaller, more manageable parts to reduce processing time and energy consumption.
-
Real-World Application: Consider the environmental implications before using ChatGPT for non-essential tasks.
-
Expert Insight: Stay updated on research regarding energy-efficient AI technologies.
Summary: The energy consumption of ChatGPT and similar LLMs is a significant concern, demanding a multi-faceted approach to mitigate its environmental impact. From optimizing algorithms to improving hardware efficiency and promoting responsible usage, concerted efforts are crucial to ensure the sustainable development of this powerful technology. The future of AI depends on addressing this challenge effectively.
Closing Message: The journey toward sustainable AI is ongoing. By understanding the environmental impact of technologies like ChatGPT, we can collectively work toward a future where powerful AI tools can coexist harmoniously with our planet. Continued research, innovative solutions, and responsible usage will be key to achieving this goal.
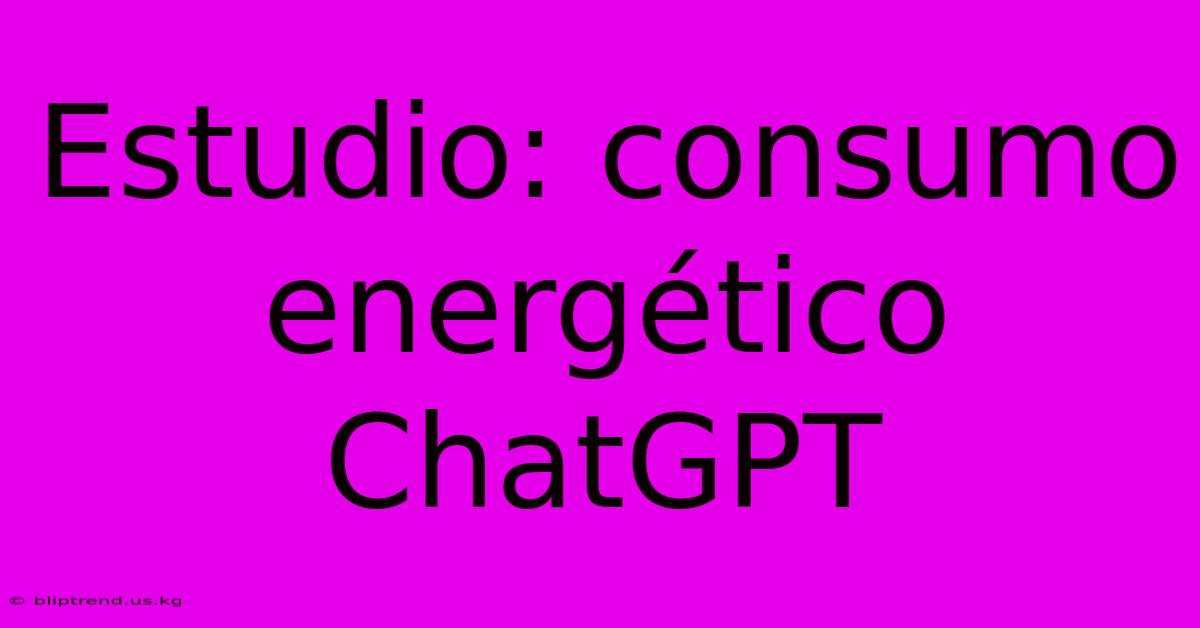
Thank you for taking the time to explore our website Estudio: Consumo Energético ChatGPT. We hope you find the information useful. Feel free to contact us for any questions, and don’t forget to bookmark us for future visits!
We truly appreciate your visit to explore more about Estudio: Consumo Energético ChatGPT. Let us know if you need further assistance. Be sure to bookmark this site and visit us again soon!
Featured Posts
-
Tupperware Feature Crossword Clue
Feb 11, 2025
-
Large Artery Crossword Clue
Feb 11, 2025
-
Take To The Limit With Out Crossword Clue
Feb 11, 2025
-
You Might Be Asking Too Much Crossword Clue
Feb 11, 2025
-
Cries Of Discovery Crossword Clue
Feb 11, 2025